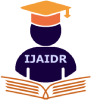
Journal of Advances in Developmental Research
E-ISSN: 0976-4844
•
Impact Factor: 9.71
A Widely Indexed Open Access Peer Reviewed Multidisciplinary Bi-monthly Scholarly International Journal
Plagiarism is checked by the leading plagiarism checker
Call for Paper
Volume 16 Issue 1
2025
Indexing Partners

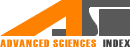
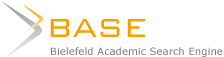

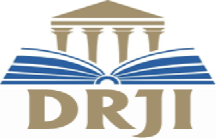

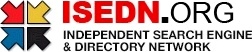

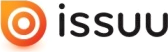

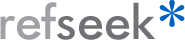
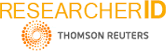
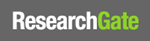
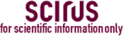
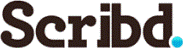

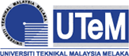
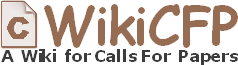
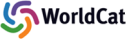
Al-Driven Privacy Audits in Adversarial Settings
Author(s) | Anshul Goel, Mangesh Pujari |
---|---|
Country | India |
Abstract | The rising number of privacy regulations forces businesses to implement AI systems for monitoring their database security. Hostile individuals have the ability to change audit data and interfere with the audit process. The study examines artificial intelligence systems that enhance privacy auditing capabilities against hostile conditions involving attackers. The current audit procedures do not adequately manage threats against data reliability as well as audit results. The research investigates innovative AI techniques to protect privacy auditors from hostile threats to their professional tasks. Current AI tools for privacy compliance receive evaluation due to their ability to identify unusual procedures and weak points in data management. Implementing machine learning algorithms in audit execution enables higher-speed quality results production. The research part analyzes how attackers exploit the vulnerabilities of machine learning to modify AI system results. As part of this research evaluation of current privacy audit frameworks and available procedures leads to developing effective AI-based privacy audit frameworks. The research produces important recommendations which cybersecurity and privacy experts need to strengthen resistance against potential attacks on audit results. Research demonstrates that AI needs to advance since it aims to protect privacy standards that accompany digital operations during present-day technological transformations. |
Keywords | AI-Driven Audits, Privacy Compliance, Adversarial Settings, Machine Learning Security, Adversarial Machine Learning, Data Protection, Privacy Auditing, Robust AI, Generative AI Risks, Privacy Governance, Cybersecurity, Data Privacy, Deep Learning Models, Explainable AI, Attack Surface, Privacy-Preserving AI, Threat Detection, Model Robustness, Secure Data Sharing, Audit Frameworks, Information Privacy, AI Ethics, Adversarial Threats, Trust In AI, Secure AI Systems, LLM Auditing, Privacy Integrity, Data Governance, Adversarial Resilience, Automated Audits |
Field | Engineering |
Published In | Volume 16, Issue 1, January-June 2025 |
Published On | 2025-01-08 |
Cite This | Al-Driven Privacy Audits in Adversarial Settings - Anshul Goel, Mangesh Pujari - IJAIDR Volume 16, Issue 1, January-June 2025. |
Share this

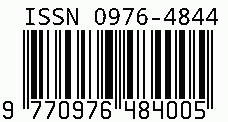
CrossRef DOI is assigned to each research paper published in our journal.
IJAIDR DOI prefix is
10.71097/IJAIDR
Downloads
All research papers published on this website are licensed under Creative Commons Attribution-ShareAlike 4.0 International License, and all rights belong to their respective authors/researchers.
